Maximizing Efficiency with an Image Classification Labeling Tool
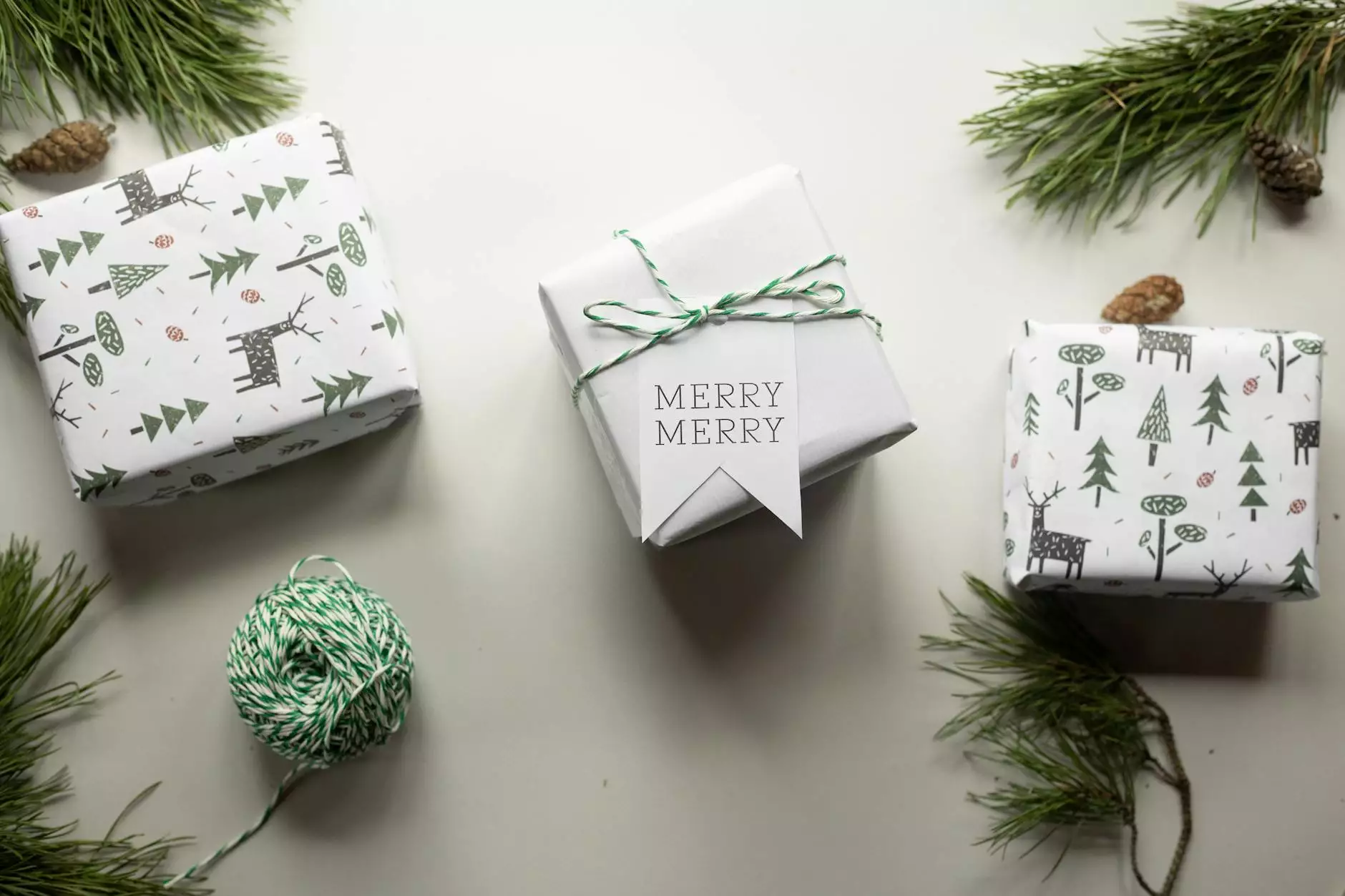
The modern era of artificial intelligence and machine learning revolves around the effectiveness and accuracy of data. As organizations continue to generate vast amounts of image data, the need for an efficient image classification labeling tool has become paramount. This article will explore the potent benefits, features, and applications of advanced data annotation platforms, particularly focusing on how they enhance image classification.
Understanding Image Classification and Its Importance
Image classification is the process of categorizing images into predefined classes or categories. It serves as a crucial component in a myriad of AI applications, from self-driving cars to medical imaging. Machine learning algorithms require large sets of labeled images for training to recognize patterns and make predictions accurately. This makes image classification labeling tools vital for:
- Automation of Data Processing: Quickens the data annotation process significantly.
- Improved Model Performance: High-quality labeled data enhances the accuracy of AI models.
- Scalability: Enables projects to scale efficiently as data volumes increase.
The Role of Data Annotation Tools
Data annotation tools are software applications designed to assist in labeling and categorizing data efficiently. These tools not only streamline the process of image labeling but also ensure precision, which is crucial for developing reliable AI models. Key features of an effective image classification labeling tool include:
1. User-Friendly Interface
A user-friendly interface is essential for both novice annotators and experienced data scientists. An intuitive layout helps reduce the learning curve and boosts productivity as users can quickly familiarize themselves with its functions.
2. Versatile Annotation Capabilities
The best tools offer a variety of annotation types such as bounding boxes, polygons, and segmentation masks. This versatility allows teams to annotate different types of images across numerous domains, enhancing the tool's utility.
3. Collaboration Features
In many organizations, data annotation is a team effort. Collaboration features enable multiple users to work on the same project simultaneously, fostering communication and improving consistency in annotations.
4. Quality Control Mechanisms
Quality assurance is paramount in data annotation. Advanced image classification labeling tools include built-in review and approval processes that ensure only high-quality annotations are used for training machine learning models.
5. Integration with Machine Learning Pipelines
Seamless integration with other AI tools and frameworks, such as TensorFlow and PyTorch, can accelerate project workflows by ensuring that annotated data can be directly employed for training AI models.
Catering to Various Industries
Data annotation is a common requirement across numerous industries. Below are some sectors that particularly benefit from image classification labeling tools:
Healthcare
In the healthcare sector, image classification can help in diagnosing diseases based on medical imaging. Annotations can guide algorithms in identifying tumors, lesions, or other anomalies, thus improving diagnostic accuracy.
Automotive
The automotive industry relies heavily on AI for autonomous vehicle technology. Image classification labeling tools help annotate images from cameras that feed into self-driving car systems, refining their ability to perceive and interpret their surroundings.
Retail
In retail, machine learning applications such as image recognition can enhance customer experience. Annotated images of products can enable visual search capabilities, helping customers find items quickly and efficiently.
Security and Surveillance
Image classification tools are instrumental in the security industry. By annotating images for facial recognition systems or anomaly detection, organizations can boost their surveillance capabilities significantly.
Best Practices for Using an Image Classification Labeling Tool
To fully leverage the potential of image classification labeling tools, organizations should adopt certain best practices, including:
1. Define Clear Objectives
Before initiating the annotation process, it’s essential to define clear objectives. Determine what types of images need to be classified and establish the categories for classification.
2. Engage Skilled Annotators
Employ skilled annotators who understand the domain of the project. Their expertise will ensure that the annotations are accurate and relevant.
3. Regular Training and Feedback
Regularly train annotators and provide feedback on their work. Continuous improvement in their annotation skills leads to better-quality outputs.
4. Utilize Automation Features
Many modern annotation tools offer automation features, such as auto-segmentation or semi-automated labeling. Utilizing these features can save time while maintaining high accuracy.
5. Monitor and Evaluate
Constantly monitor the annotation process and evaluate the quality of the labeled data. Implement checks and audits to ensure adherence to quality standards.
Choosing the Right Image Classification Labeling Tool
When selecting an image classification labeling tool, consider the factors that are most relevant to your organization's needs:
- Features: Ensure the tool has robust features that meet your annotation requirements, such as collaboration, quality control, and integration capabilities.
- Scalability: Choose a tool that grows with your needs. As your dataset expands, the tool should accommodate increased annotation volume without compromising quality.
- Customer Support: Reliable customer support can ease the transition to new tools and help resolve any challenges quickly.
- Pricing: Assess the pricing structure to find a solution that provides the best return on investment. Consider both short-term and long-term costs associated with the tool.
Conclusion
In conclusion, the future of artificial intelligence relies heavily on the quality of data, with image classification labeling tools playing a central role in enhancing this quality. By leveraging these tools effectively, organizations can significantly improve their data annotation processes, leading to more accurate machine learning models and better outcomes across various industries. Embracing the right data annotation tool not only enhances workflow efficiency but also positions organizations at the forefront of technological advancement.
For businesses looking to optimize their data annotation processes, consider exploring the comprehensive solutions offered by Keylabs.ai. With a focus on quality, efficiency, and user experience, the right labeling tool can propel your data initiatives forward.