Machine Learning Annotation Tools: Empowering Software Development
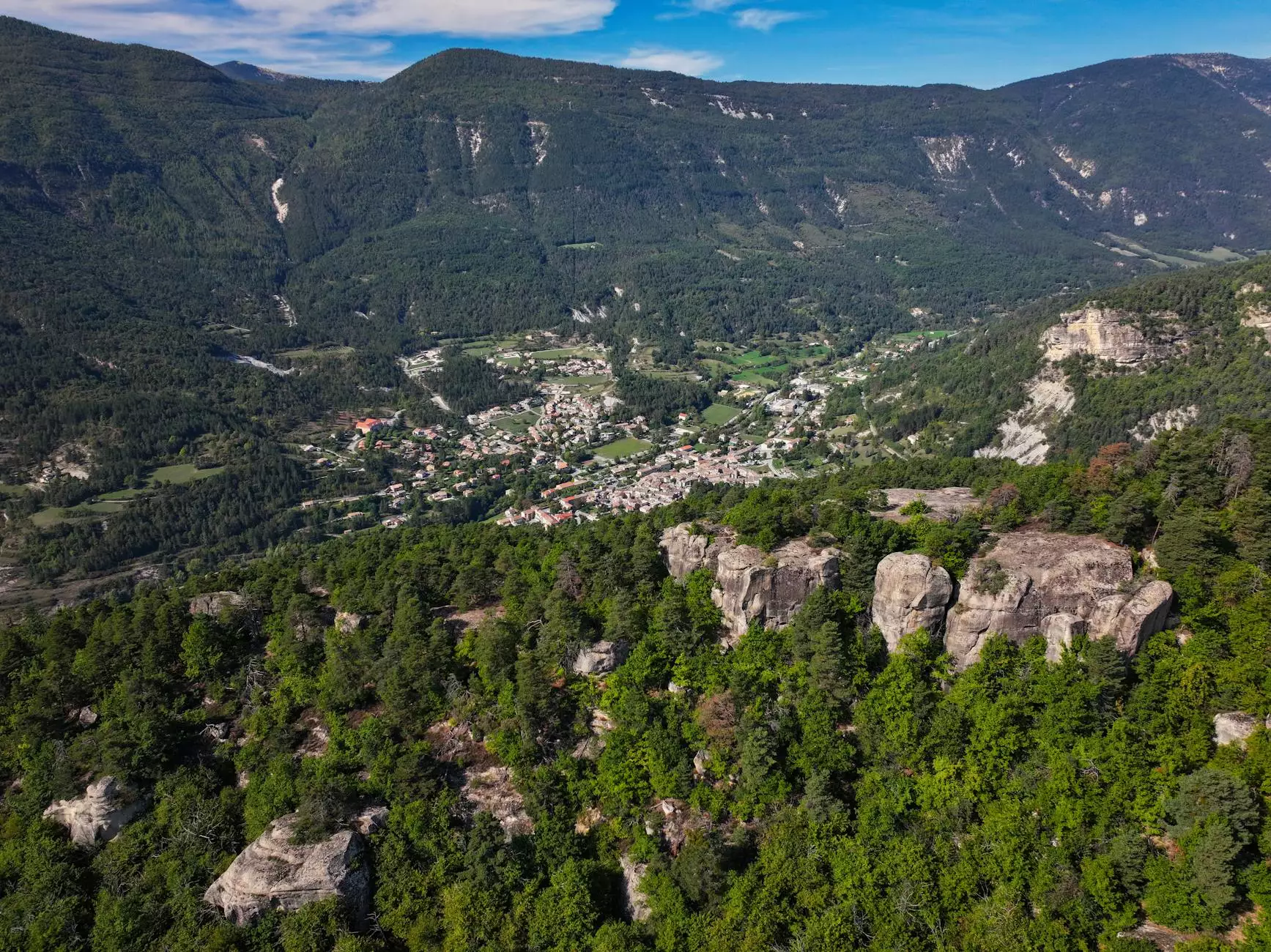
The landscape of artificial intelligence (AI) and machine learning (ML) is evolving rapidly, bringing unprecedented changes to the way businesses operate. At the core of these advancements lies a crucial process: data annotation. This article dives deep into the world of machine learning annotation tools, their significance in software development, and how they can revolutionize the efficiency and effectiveness of AI projects.
Understanding Machine Learning Annotation Tools
Machine learning algorithms require high-quality data to train and make accurate predictions. This is where machine learning annotation tools come into play. These tools are designed to label, categorize, and organize data, facilitating the machine learning process. Data can be anything from images and text to audio and video, and proper annotation is essential for training models effectively.
The Importance of Data Annotation
The act of annotating data ensures that a machine learning model understands the context and nuances of the information it processes. Whether it’s identifying objects in images or categorizing text, proper annotation enhances model accuracy. The importance of data annotation can be summarized in the following points:
- Improves Model Performance: Well-annotated data leads to more accurate and robust machine learning models.
- Reduces Bias: Diverse labeling helps reduce bias in ML models, creating fairer outcomes.
- Saves Time: Automated annotation tools can significantly speed up the data preparation process.
- Enhances Collaboration: Standardized annotation fosters better teamwork among data scientists and developers.
Types of Machine Learning Annotation Tools
There are several types of machine learning annotation tools available, each serving a unique purpose. The selection of the right tool depends on the data type and the specific project requirements. Here are some common types:
1. Image Annotation Tools
Image annotation tools help label images for tasks such as object detection, segmentation, and classification. Popular techniques used in these tools include:
- Bounding Boxes: Used to encase objects within an image.
- Polygon Annotation: Allows for precise representation of object shapes.
- Landmark Annotation: Focuses on specific points of an object in an image.
2. Text Annotation Tools
Text annotation tools are valuable for natural language processing (NLP) tasks. They provide functionalities like:
- Sentiment Analysis: Identifying the sentiment of a given text.
- Named Entity Recognition: Detecting proper names within text.
- Part-of-Speech Tagging: Marking words according to their grammatical role.
3. Audio and Video Annotation Tools
These tools help in annotating audio tracks and video files, useful for applications like speech recognition and video classification. They include features for:
- Transcribing: Converting speech into written text.
- Action Recognition: Identifying actions happening within a video scene.
- Audio Classification: Categorizing different sound types in recordings.
Challenges in Data Annotation
While annotation is vital, it’s not without its challenges. Businesses often face issues such as:
1. Time-Consuming Process
Data annotation can be a labor-intensive task, particularly if done manually. Depending on the project's scope, it can take a considerable amount of time to gather and annotate enough data for high-quality machine learning models.
2. Quality Control
Ensuring the quality and accuracy of annotations is crucial. Poorly labeled data can lead to misleading model results, which may ultimately impact business decisions.
3. Scaling Challenges
As the volume of data grows, so does the need for efficient annotation processes. Scaling manual efforts can be cumbersome, and without the right tools, organizations risk falling behind.
How to Choose the Right Machine Learning Annotation Tool
Selecting the right machine learning annotation tool for your business can significantly impact the success of your AI initiatives. Here are key considerations:
1. Data Type Compatibility
Make sure the tool you choose supports the type of data you’re working with, be it images, text, audio, or video.
2. User-Friendliness
Look for tools that offer intuitive interfaces to streamline the annotation process, minimizing the learning curve for your team.
3. Automation Features
Consider tools with automation capabilities, such as pre-trained models that can expedite the annotation process and reduce labor costs.
4. Scalability
Choose tools that can easily scale as your data requirements grow. This prevents the need for continual re-evaluation of your tech stack.
5. Integration
Ensure that the annotation tool can integrate seamlessly with your existing software development environment and machine learning frameworks.
The Future of Machine Learning Annotation Tools
As AI technology continues to advance, the role of machine learning annotation tools will become even more critical. The future trends within this domain include:
1. Enhanced Automation
With advancements in AI and ML, tools are becoming smarter, incorporating capabilities for automated annotation that improve speed and accuracy.
2. Collaborative Annotation Platforms
Emerging tools are focusing on enhancing collaboration among teams through cloud-based platforms, allowing for real-time updates and communication.
3. Integration with Advanced AI
Future annotation tools will likely integrate advanced AI features directly into the annotation process, allowing for predictive suggestions and auto-corrections.
4. Improved Quality Control Mechanisms
Enhanced systems for quality assurance in annotation will help reduce human errors, ensuring that the data is of the highest quality.
Conclusion: Transforming Your Business with Machine Learning Annotation Tools
The right machine learning annotation tools can significantly transform how businesses leverage data in AI initiatives. By understanding the intricacies of data annotation, selecting the appropriate tools, and embracing best practices, companies can propel their software development efforts forward, leading to better outcomes and innovations. As AI continues to shape the future of industries worldwide, investing in quality annotation tools is not just an option—it’s a necessity for companies looking to stay ahead.
For more information on how machine learning annotation tools can enhance your software development processes, consider exploring the offerings available at keymakr.com.